Featured Book
Summary of Kahneman’s Thinking, Fast and Slow
By Daniel Kahneman
Review by Mark Looi (republished from marklooi.medium.com)
Daniel Kahneman is a Nobel Laureate in Economics who is a psychologist by training. He won the prize mostly for his work in decision making, specifically Prospect Theory. This book distills a lifetime of work on the engine of human thinking, highlighting our cognitive biases and showing both the brilliance and limitations of the human mind. This summary attempts to capture some of the more interesting findings.
(Excerpts and quotes are from: Daniel Kahneman. Thinking, Fast and Slow. Apple Books.)
Kahneman writes the book as a lay person’s introduction to experimental psychology and summarizes some of the major results of the past 40 years. In doing so, he gives a high level description of the scientific method as applied in social science, the art of creating hypotheses, the clever experiments to test them, and a little about how the data are analyzed. He shows how slowly but surely, in conjunction with many researchers around the globe, our understanding of human thinking has advanced.
He also recounts the impressive history of the field, going back to great rational thinkers, Bernoulli (of the famous Bernoulli Equation) and David Hume, the Scottish philosopher.
In the end, Kahneman shows that our brains are highly evolved to perform many tasks with great efficiency, but they are often ill-suited to accurately carry out other mental tasks; in fact, our thinking is riddled with behavioral fallacies. Consequently, we are at risk of manipulation not usually of the overt kind, but by nudges and small increments. Indeed we have learned that by exploiting these weaknesses in the way our brains process information, social media platforms, governments, media in general, and populist leaders, are able exercise a form of collective mind control.
It’s also clear that the bugs in our personal thinking systems are being exploit faster than patches can be applied!
Two Systems
Kahneman introduces two characters that animate the mind:
- “System 1 operates automatically and quickly, with little or no effort and no sense of voluntary control.
- System 2 allocates attention to the effortful mental activities that demand it, including complex computations. The operations of System 2 are often associated with the subjective experience of agency, choice, and concentration.”
These two systems somehow co-exist in the human brain and together help us navigate life; they aren’t literal or physical, but conceptual. System 1 is an intuitive system that cannot be turned off; it helps us perform most of the cognitive tasks that everyday life requires, such as identify threats, navigate our way home on familiar roads, know that 2+2=4, recognize friends, and so on. System 2 can help us analyze complex problems, do math exercises, do crossword puzzles, and so on. Even though System 2 is useful, it takes effort and energy to engage it. So, it tends to take shortcuts at the behest of System 1. For example, the syllogism,
- All roses are flowers.
- Some flowers fade quickly.
- Therefore, some roses fade quickly.
is considered by a large majority of college students to be correct. Of course, it isn’t. We get fooled because intuitively we know that roses fade. But this syllogism is not a statement about the world; it’s about logical relationships. The energy required by System 2 to fully analyze the statements is relatively high; System 1 jumps to the conclusion that the conclusion is true and convinces System 2. It turns out that when people first come to believe a false statement, they are very likely to believe arguments that support it; this is the basis for confirmation bias.
According to Kahneman, these are the “Characteristics of System 1:
- generates impressions, feelings, and inclinations; when endorsed by System 2 these become beliefs, attitudes, and intentions
- operates automatically and quickly, with little or no effort, and no sense of voluntary control
- can be programmed by System 2 to mobilize attention when a particular pattern is detected (search)
- executes skilled responses and generates skilled intuitions, after adequate training
- creates a coherent pattern of activated ideas in associative memory
- links a sense of cognitive ease to illusions of truth, pleasant feelings, and reduced vigilance
- distinguishes the surprising from the normal
- infers and invents causes and intentions
- neglects ambiguity and suppresses doubt
- is biased to believe and confirm
exaggerates emotional consistency (halo effect) - focuses on existing evidence and ignores absent evidence (WYSIATI)
generates a limited set of basic assessments - represents sets by norms and prototypes, does not integrate
- matches intensities across scales (e.g., size to loudness)
- computes more than intended (mental shotgun)
- sometimes substitutes an easier question for a difficult one (heuristics)
- is more sensitive to changes than to states (prospect theory)*
- overweights low probabilities*
- shows diminishing sensitivity to quantity (psychophysics)*
- responds more strongly to losses than to gains (loss aversion)*
- frames decision problems narrowly, in isolation from on another”
What now follows are a summary of the major fallacies that Kahneman identifies.
Priming
Our minds are wonderful associative machines, allowing us to easily associate words like “lime” with “green”. Because of this, we are susceptible to priming, in which a common association is invoked to move us in a particular direction or action. This is the basis for “nudges” and advertising using positive imagery.
Cognitive Ease
Whatever is easier for System 2 is more likely to be believed. Ease arises from idea repetition, clear display, a primed idea, and even one’s own good mood. It turns out that even the repetition of a falsehood can lead people to accept it, despite knowing it’s untrue, since the concept becomes familiar and is cognitively easy to process.
Jumping to Conclusions
Our System 1 is “a machine for jumping to conclusions” by basing its conclusion on “What You See Is All There Is” (WYSIATI). WYSIATI is the tendency for System 1 to draw conclusions based on the readily available, sometimes misleading information and then, once made, to believe in those conclusions fervently. The measured impact of halo effects, confirmation bias, framing effects, and base-rate neglect are aspects of jumping to conclusions in practice. One example is confirmation bias, where we are more open to and looking for evidence that supports our beliefs, rather than what doesn’t. Rationally, we should look for evidence that contradicts beliefs since that will subject our belief system to greater scrutiny. But outside of the rigors of pure science, such an approach is uncommon. (In the sciences, one methodology is to construct a so-called null hypothesis, the reject of which proves the original claim.)
Answering an Easier Question
Often when dealing with a complex or difficult issue, we transform the question into an easier one that we can answer. In other words, we use a heuristic; for example, when asked “How happy are you with life”, we answer the question, “What is my mood now”. While these heuristics (which enjoys the same root as the word “eureka”) can be useful, they often lead to incorrect conclusions.
Law of Small Numbers
We have an exaggerated faith in small samples, but our tendency to seek patterns and explanation leads us to a causal explanation of chance events that are wrong or unsupportable. Even researchers like Kahneman himself fall prey to the inadequacy of sample size in their research.
Anchors
Anchoring is a form of priming the mind with an expectation. An example are the questions: “Is the height of the tallest redwood more or less than x feet? What is your best guess about the height of the tallest redwood?” When x was 1200, answers to the second question was 844; when x was 180, the answer was 282.
Availability
The bias of Availability occurs when we take into account a salient event, a recent experience, or something that’s particularly vivid to us, to make our judgments. People who are guided by System 1 are more susceptible to the Availability bias than others; in particular:
- when they are engaged in another effortful task at the same time
- when they are in a good mood because they just thought of a happy episode in their life
- if they score low on a depression scale
- if they are knowledgeable novices on the topic of the task, in contrast to true experts
- when they score high on a scale of faith in intuition
- if they are (or are made to feel) powerful
Representativeness
Representativeness is where we use stereotypes to help us judge probabilities. For example, “you see a person reading The New York Times on the subway. Which of the following is a better bet about the reading stranger? 1) She has a PhD. 2) She does not have a college degree.” The sin of representativeness is where we might pick the second answer, even though the probability of PhDs on the subway is far less than people without degrees. Though a simple example, one way to resist the temptation of representativeness is to consider the base rate (in this case, the rate of PhDs vs. non-PhDs) and make the judgment from that.
Less Is More
Given the description, “Linda is thirty-one years old, single, outspoken, and very bright. She majored in philosophy. As a student, she was deeply concerned with issues of discrimination and social justice, and also participated in anti-nuclear demonstrations. Which alternative is more probable?
- Linda is a bank teller.
- Linda is a bank teller and is active in the feminist movement.”
In this case, the additional detail that Linda is “active in the feminist movement” in answer 2., only serves to make the probability lower, since it imposes more constraints. But, because of the accompanying narrative, we like the second option, even though it is less likely. This is why Less is More.
Causes Trump Statistics
The finding from a number of researchers is that people are poor statistical reasoners and they have limited ability to think in Bayesian terms, even when supplied with obviously relevant background data. Bayesian inference is the widely used method to reason about likelihoods given a prior known condition. For example, he uses the example:
“A cab was involved in a hit-and-run accident at night. Two cab companies, the Green and the Blue, operate in the city.
- 85% of the cabs in the city are Green and 15% are Blue.
- A witness identified the cab as Blue. The court tested the reliability of the witness under the circumstances that existed on the night of the accident and concluded that the witness correctly identified each one of the two colors 80% of the time and failed 20% of the time.
What is the probability that the cab involved in the accident was Blue rather than Green?”
Apparently a lot of people ignore the first fact, which defines the base rate of Green and Blue cabs. Kahneman doesn’t go into much detail about how to make the calculations, but it is an application of Bayes’ Rule. To wit,
A = Cab is blue, B = Cab is identified as blue; therefore, ⌐A = Cab is green, ⌐B = Cab is identified as green. So, we have:
P(A) = 0.15, P(⌐A) = 0.85, P(B|A) = 0.8, P(⌐B|⌐A) = 0.8, P(B|⌐A)= 0.2, P(⌐B|A) = 0.2
Thus, we want to know, P(A|B) = P(B|A)*P(A)/P(B), i.e., the probability that the cab was blue rather than green (and mistakenly identified).
And, we know from the Theorem of Total Probability that P(B) = P(B|A)*P(A) + P(B|⌐A)*P*(⌐A). Therefore, substituting, we get:
0.8*0.15/[0.8*0.15 + 0.2 *0.85] = 0.41, or 41%.
This Bayesian reasoning comes up in many practical situations, such as calculating medical diagnosis of an individual, where there is a base rate of a disease in a population and a test which is, for example, 95% effective at identifying the disease.
Kahneman quotes two famous social scientists (Nisbett and Borgida):
“Subjects’ unwillingness to deduce the particular from the general was matched only by their willingness to infer the general from the particular.”
Regression to the Mean
Regression to the mean is the statistical fact that any sequence of trials will eventually converge to the expected value (i.e., the mean). Unfortunately, we often look for causal reasons to explain lucky streaks and other sequences of seemingly meaningful numbers. When further embellished by other details like a “hot hand”, we tend to find causal explanations.
Kahneman goes on to describe still more mental shortcomings, such as:
- Illusion of understanding: we construct narratives to aid in understanding and to make sense of the world. We look for causality where none exists.
- Illusion of validity: pundits, stock pickers and other experts develop an outsized sense of expertise.
- Expert intuition: algorithms, even seemingly primitive ones, applied with discipline often outdo experts.
- Planning fallacy: this fallacy afflicts many professions and stem from plans and forecasts that are unrealistically close to best case; and, do not take into account the actual results of similar projects.
- Optimism and the Entrepreneurial Delusion: most people are overconfident, tend to neglect competitors, and believe they will outperform the average.
Bernoulli, Expected Utility and Prospect Theory
Kahneman criticizes Bernoulli, who nearly 250 years ago propounded Utility Theory, which in essence explains people’s choices and motivations by the utility of the outcomes. But choices were not just the mathematically determined expected value, but on a psychological value, the utility. Here, people act in risk averse ways, preferring sure bets to risks, even bets that are mathematically equivalent (e.g., winning $500 outright; or a 50% chance at $1000). Further, utility is relative to the wealth or poverty of the individual. And, it explains why all other things equal, a poorer person will buy insurance to transfer the risk of loss to a richer one. So far, so good.
However, Kahneman points out that Bernoulli’s theory breaks down because it doesn’t take into account the initial reference state. For example,
“Anthony’s current wealth is 1 million. Betty’s current wealth is 4 million.
They are both offered a choice between a gamble and a sure thing.
The gamble: equal chances to end up owning 1 million or 4 million; or, the sure thing: own 2 million for sure.
In Bernoulli’s account, Anthony and Betty face the same choice: their expected wealth will be 2.5 million if they take the gamble and 2 million if they prefer the sure-thing option. Bernoulli would therefore expect Anthony and Betty to make the same choice, but this prediction is incorrect. Here again, the theory fails because it does not allow for the different reference points from which Anthony and Betty consider their options.”
Betty stands to lose a lot of her wealth and will be unhappy regardless. Anthony is elated because he gains, also regardless.
“In Bernoulli’s theory you need to know only the state of wealth to determine its utility, but in prospect theory you also need to know the reference state,” that is, the initial conditions. They also describe the loss aversion of most people and when confronted with the prospect of losses, people will take on more risk in an effort to avoid the loss, even if mathematically, they would be no better or even worse off. This explains why people caught in desperate situations seem to engage in riskier behavior: “people who face very bad options take desperate gambles, accepting a high probability of making things worse in exchange for a small hope of avoiding a large loss.”
Endowment Effect
Most people are familiar with one aspect of the endowment effect, the sunk cost fallacy. With experience and training, people like traders can overcome the sunk cost or endowment effect. The key difference seems to be whether or not goods are held for trading or for use. In the latter case, the sunk cost or endowment effects are larger.
Loss Aversion
Another measured phenomenon is loss aversion. It permeates much of life, including regulations and reforms that make remove benefits from one group in favor of another, even though it may result in an overall increase in utility.
People Aren’t Rational
The standard treatment of actors in economics is to assume rationality. But, it turns out people are not entirely rational. They generally prefer sure things; they have a propensity to value the elimination of risk over that of rationally reducing it to an acceptable level. People attach value to gains and losses (i.e., the change) rather than to wealth itself.
The Fourfold Pattern
Prospect Theory is summarized in the following table:
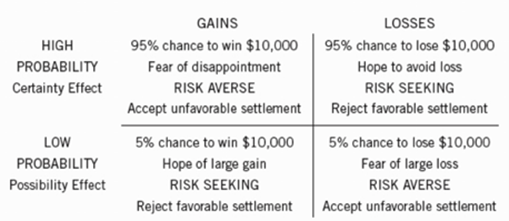
Kahneman says it best:
- “The top left is the one that Bernoulli discussed: people are averse to risk when they consider prospects with a substantial chance to achieve a large gain. They are willing to accept less than the expected value of a gamble to lock in a sure gain.
- The possibility effect in the bottom left cell explains why lotteries are popular. When the top prize is very large, ticket buyers appear indifferent to the fact that their chance of winning is minuscule. …
- The bottom right cell is where insurance is bought. People are willing to pay much more for insurance than expected value — which is how insurance companies cover their costs and make their profits. … they eliminate a worry and purchase peace of mind. …
- Many unfortunate human situations unfold in the top right cell. This is where people who face very bad options take desperate gambles, accepting a high probability of making things worse in exchange for a small hope of avoiding a large loss. Risk taking of this kind often turns manageable failures into disasters. The thought of accepting the large sure loss is too painful, and the hope of complete relief too enticing, to make the sensible decision that it is time to cut one’s losses. This is where businesses that are losing ground to a superior technology waste their remaining assets in futile attempts to catch up. Because defeat is so difficult to accept, the losing side in wars often fights long past the point at which the victory of the other side is certain, and only a matter of time.”
Frames of Reference
How a problem is framed makes a big difference in perceptions and solutions. He illustrates it with the famous MPG Illusion. “Consider two car owners who seek to reduce their costs:
- Adam switches from a gas-guzzler of 12 mpg to a slightly less voracious guzzler that runs at 14 mpg.
- The environmentally virtuous Beth switches from a 30 mpg car to one that runs at 40 mpg.
Suppose both drivers travel equal distances over a year. Who will save more gas by switching? You almost certainly share the widespread intuition that Beth’s action is more significant than Adam’s: she reduced mpg by 10 miles rather than 2, and by a third (from 30 to 40) rather than a sixth (from 12 to 14). Now engage your System 2 and work it out. If the two car owners both drive 10,000 miles, Adam will reduce his consumption from a scandalous 833 gallons to a still shocking 714 gallons, for a saving of 119 gallons. Beth’s use of fuel will drop from 333 gallons to 250, saving only 83 gallons. The mpg frame is wrong, and it should be replaced by the gallons-per-mile frame (or liters-per–100 kilometers, which is used in most other countries). As Larrick and Soll point out, the misleading intuitions fostered by the mpg frame are likely to mislead policy makers as well as car buyers.”
Overweighting the Recent
People tend to overweight recent experiences and the positive or negative perception of one is disproportionately determined by the last episodes of the entire experience. So, a vacation that starts out badly but has a pleasant ending is likely to be remembered favorably; the opposite sequence may undermine the overall experience, even if objectively the bad parts were of no greater duration in either case.
Conclusion
Kahneman’s book is an important summary for the general reader of the advances in behavioral psychology in the past 40 years. The main criticism could be that he split hairs and applies a precise interpretation to questions like the Linda problem which normal people in everyday life would not. In fact, people use their contextual and cultural knowledge to form insights that go beyond the obvious facts of the case. This would be the simplest most sympathetic explanation of the Linda problem or Less is More. Indeed, parsing statements too precisely is often considered a faux pas or a suggestion of a lack of social skills. For example, taking to task someone for using the word “literally” for “figuratively” seems pedantic today. Yet, this is the nature of science: to ask precise questions so as to successively narrow down what remains ambiguous.
Kahneman shows the rational animal favored by Plato, Aristotle, and the Enlightenment, in a different light: a product of our evolutionary environment and in many ways ill-equipped to deal with a rational, science-based, logical world. Worse, we are at constant risk of repeating the same cognitive errors and biases, easily manipulated, and riven by irrational beliefs and fears. In a reality that’s dominated by science and statistics, most of humankind lacks the basic knowledge and experience to thrive. In fact, a tiny minority with those capabilities are able to manipulate the others and command great wealth.